النبات
مواضيع عامة في علم النبات
الجذور - السيقان - الأوراق
النباتات الوعائية واللاوعائية
البذور (مغطاة البذور - عاريات البذور)
الطحالب
النباتات الطبية
الحيوان
مواضيع عامة في علم الحيوان
علم التشريح
التنوع الإحيائي
البايلوجيا الخلوية
الأحياء المجهرية
البكتيريا
الفطريات
الطفيليات
الفايروسات
علم الأمراض
الاورام
الامراض الوراثية
الامراض المناعية
الامراض المدارية
اضطرابات الدورة الدموية
مواضيع عامة في علم الامراض
الحشرات
التقانة الإحيائية
مواضيع عامة في التقانة الإحيائية
التقنية الحيوية المكروبية
التقنية الحيوية والميكروبات
الفعاليات الحيوية
وراثة الاحياء المجهرية
تصنيف الاحياء المجهرية
الاحياء المجهرية في الطبيعة
أيض الاجهاد
التقنية الحيوية والبيئة
التقنية الحيوية والطب
التقنية الحيوية والزراعة
التقنية الحيوية والصناعة
التقنية الحيوية والطاقة
البحار والطحالب الصغيرة
عزل البروتين
هندسة الجينات
التقنية الحياتية النانوية
مفاهيم التقنية الحيوية النانوية
التراكيب النانوية والمجاهر المستخدمة في رؤيتها
تصنيع وتخليق المواد النانوية
تطبيقات التقنية النانوية والحيوية النانوية
الرقائق والمتحسسات الحيوية
المصفوفات المجهرية وحاسوب الدنا
اللقاحات
البيئة والتلوث
علم الأجنة
اعضاء التكاثر وتشكل الاعراس
الاخصاب
التشطر
العصيبة وتشكل الجسيدات
تشكل اللواحق الجنينية
تكون المعيدة وظهور الطبقات الجنينية
مقدمة لعلم الاجنة
الأحياء الجزيئي
مواضيع عامة في الاحياء الجزيئي
علم وظائف الأعضاء
الغدد
مواضيع عامة في الغدد
الغدد الصم و هرموناتها
الجسم تحت السريري
الغدة النخامية
الغدة الكظرية
الغدة التناسلية
الغدة الدرقية والجار الدرقية
الغدة البنكرياسية
الغدة الصنوبرية
مواضيع عامة في علم وظائف الاعضاء
الخلية الحيوانية
الجهاز العصبي
أعضاء الحس
الجهاز العضلي
السوائل الجسمية
الجهاز الدوري والليمف
الجهاز التنفسي
الجهاز الهضمي
الجهاز البولي
المضادات الحيوية
مواضيع عامة في المضادات الحيوية
مضادات البكتيريا
مضادات الفطريات
مضادات الطفيليات
مضادات الفايروسات
علم الخلية
الوراثة
الأحياء العامة
المناعة
التحليلات المرضية
الكيمياء الحيوية
مواضيع متنوعة أخرى
الانزيمات
Secondary Structure Predictions
المؤلف:
Robert Schleif
المصدر:
Genetics and Molecular Biology
الجزء والصفحة:
2nd Edition , p168-170
2025-05-10
197
Less ambitious than calculating the tertiary structure of a protein is predicting its secondary structure. There is some hope that this is a much simpler problem than prediction of tertiary structure because most of the interactions determining secondary structure at an amino acid residue derive from amino acids close by in the primary sequence. The problem is how many amino acids need to be considered and how likely is a prediction to be correct? We can estimate both by utilizing information from proteins whose structures are known. The question is how long a stretch of amino acids is required to specify a secondary structure. For example, if a stretch of five amino acids were sufficient, then the same sequence of five amino acids ought to adopt the same structure, regardless of the protein in which they occur.
The tertiary structures available from X-ray diffraction studies can be used as input data. Many examples now exist where a sequence of five amino acids appears in more than one protein. In about 60% of these cases, the same sequence of five amino acids is found in the same secondary structure. Of course, not all possible five amino acid sequences are represented in the sample set, but the set is sufficiently large that it is clear that we should not expect to have better than about 60% accuracy in any secondary structure prediction scheme if we consider only five amino acids at a time.
Several approaches have been used to determine secondary structure prediction rules. At one end is a scheme based on the known conformations assumed by homopolymers and extended by analysis of a small number of known protein structures. The Chou and Fasman approach is in this category. A more general approach is to use information theory to generate a defined algorithm for predicting secondary structure. This overcomes many of the ambiguities of the Chou-Fasman prediction scheme.
Recently, neural networks have been applied to predicting secondary structure. While usually implemented on ordinary computers, these simulate on a crude scale some of the known properties of neural connections in parts of the brain. Depending on the sum of the positive and negative inputs, a neuron either does not fire, or fires and sends activating and inhibiting signals on to the neurons its output is connected to.
In predicting secondary structure by a neural network, the input is the identity of each amino acid in a stretch of ten to fifteen amino acids (Fig. 1). Since each of these can be any of the twenty amino acids, about 200 input lines or “neurons” are on this layer. Each of these activates or inhibits each neuron on a second layer by a strength that is adjusted by training. After summing the positive and negative signals reaching it, a neuron on the second layer either tends to “fire” and sends a strong activating or inhibiting signal on to the third layer, or it tends not to fire. In the case of protein structure prediction, there would be three neurons in the third layer. One corresponds to predicting α-helix, one to β-sheet, and one to random coil. For a given input sequence, the network’s secondary structure prediction for the central amino acid of the sequence is considered to correspond to the neuron of the third layer with the highest output value. “Training” such a network is done by presenting various stretches of amino acids whose secondary structure is known and adjusting the strengths of the interactions between neurons so that the network predicts the structures correctly.
Fig1. A three layer neural network of 20 × 13 inputs, a middle layer, and an output layer. Each of the input "neurons" is connected to each of the middle layer neurons and each of the middle layer neurons is connected to the three output neurons. The strengths of the interactions are not all equal.
No matter what scheme is used, the accuracy of the resulting structure prediction rules never exceeds about 65%. Note that a scheme with no predictive powers whatsoever would be correct for about 33% of the amino acids in a protein. The failure of these approaches to do better than 65% means that in some cases, longer-range interactions between amino acids in a protein have a significant effect in determining secondary structure.
الاكثر قراءة في مواضيع عامة في الاحياء الجزيئي
اخر الاخبار
اخبار العتبة العباسية المقدسة
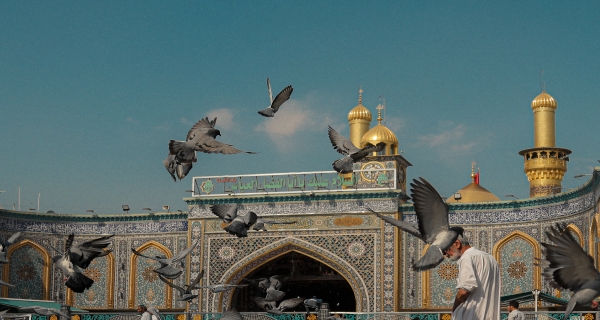
الآخبار الصحية
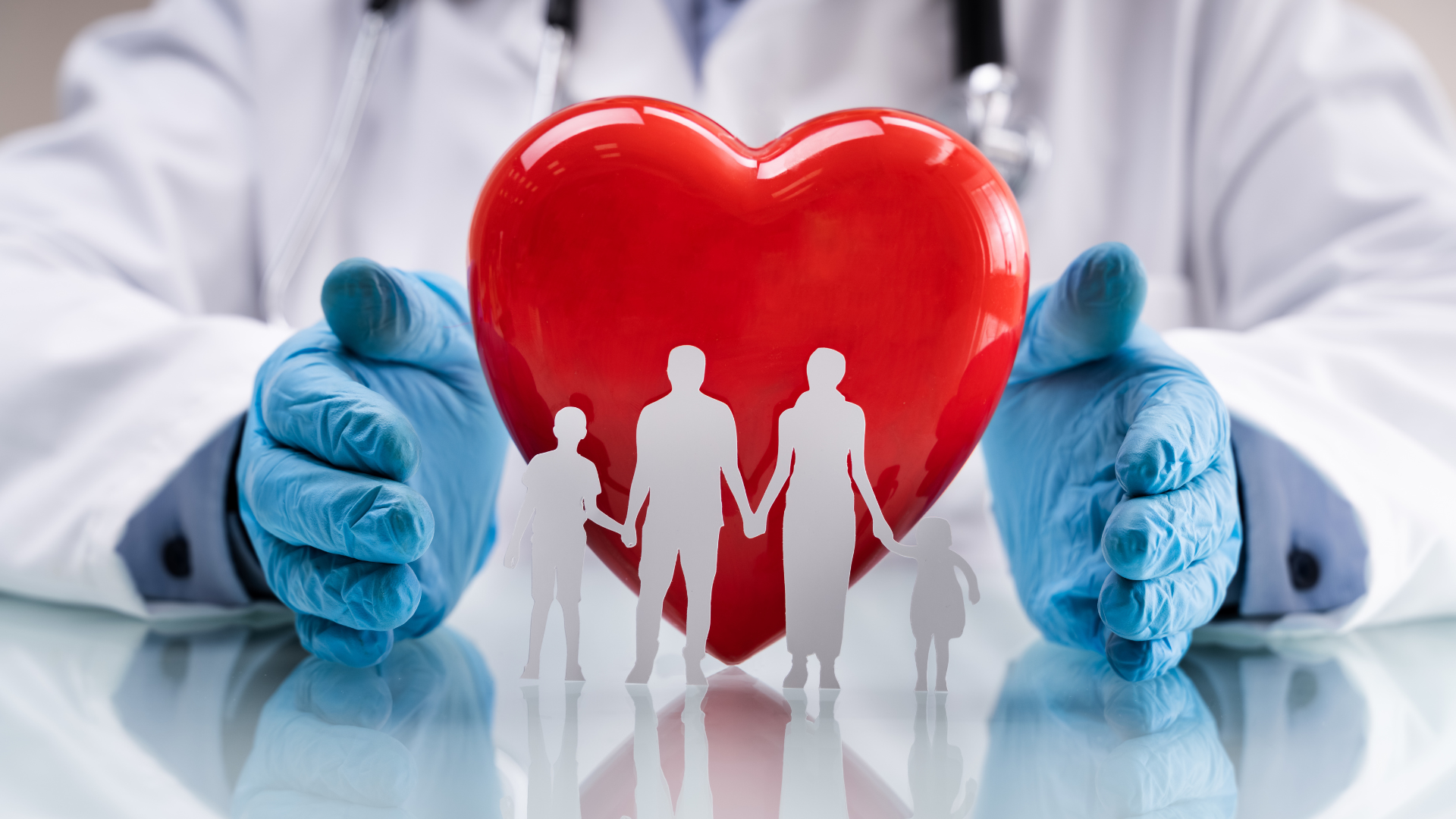